Understanding Agentic AI
Enhancing Supply Chain Resilience
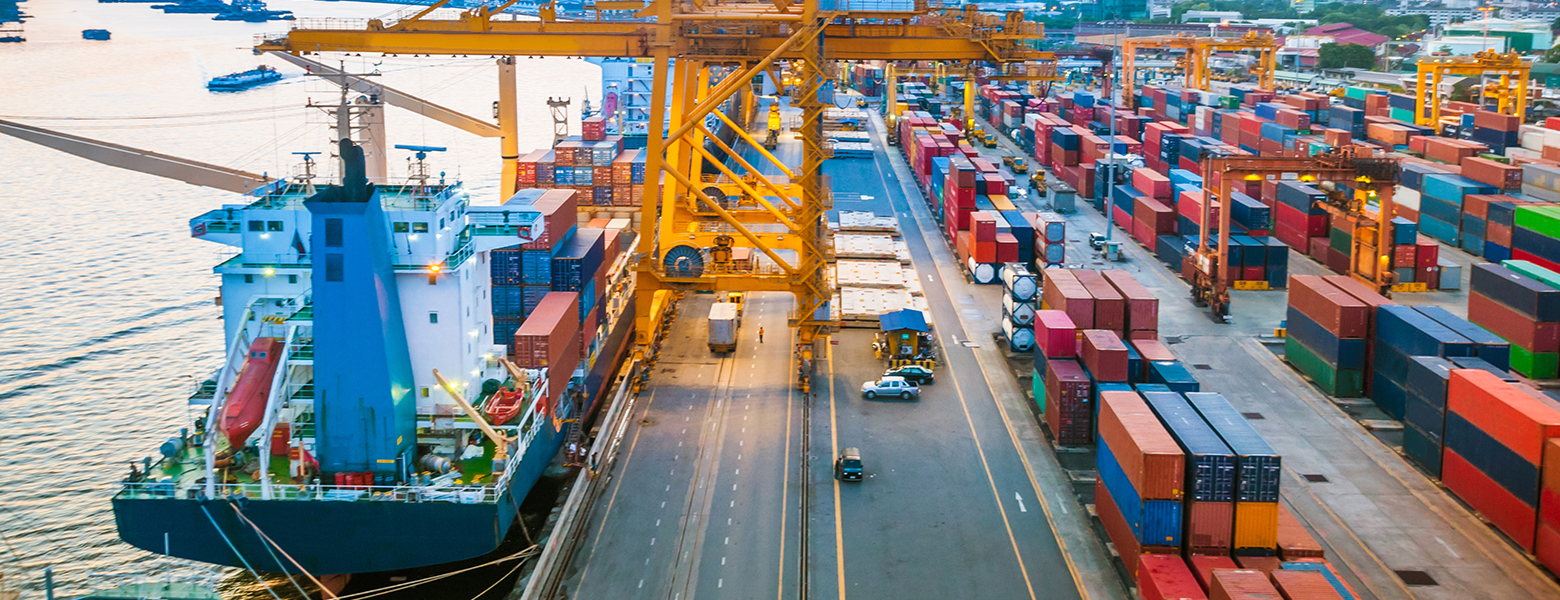
Demand Forecasting and Inventory Management
- Case Study: A manufacturer producing electronic components for electric vehicles uses agentic AI to predict surges in demand during new model launches. The system autonomously adjusts inventory levels by increasing production schedules and optimizing raw material procurement ahead of time. This proactive approach ensures that the manufacturer can meet JIT requirements without delays.
Real-Time Monitoring and Adaptability
- Case Study: An auto-component supplier experiences a sudden disruption due to a factory shutdown caused by flooding. The agentic AI detects this disruption in real-time and automatically reroutes shipments from alternative suppliers while adjusting production schedules to mitigate impacts on delivery timelines.
Optimizing Logistics and Route Planning
- Case Study: A manufacturer of automotive parts leverages agentic AI to optimize its logistics network. When severe weather is forecasted, the system autonomously reroutes deliveries away from affected areas and adjusts transportation schedules accordingly. As a result, the manufacturer minimizes delays and ensures that components arrive at assembly plants on time.
Supplier Relationship Management
- Case Study: A manufacturer integrates agentic AI into their supply chain process for supplier relationship management. The AI agents autonomously assess supplier performance, monitor compliance, and ensure that materials are delivered on time. This enhances collaboration with suppliers and reduces the risk of delays in production processes.
Risk Management
- Case Study: During the COVID-19 pandemic, many manufacturers faced significant supply chain disruptions. Companies utilizing agentic AI were able to predict shortages by analyzing global shipping trends and local market conditions, allowing them to implement contingency plans proactively.
Challenges Addressed by Agentic AI
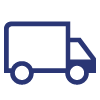
Complexity of Global Supply Chains
Traditional supply chain management often struggles with the intricacies of global logistics networks. Agentic AI simplifies this complexity by providing real-time analytics that enhance decision-making.
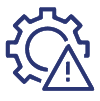
Risk Management
By predicting potential disruptions through advanced analytics, agentic AI empowers manufacturers to implement contingency plans before issues escalate.
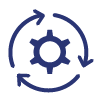
Resource Optimization
With its ability to autonomously adjust inventory levels and production schedules based on real-time data, agentic AI helps manufacturers reduce excess stock and avoid stockouts.
Challenges in Building Agentic AI Systems
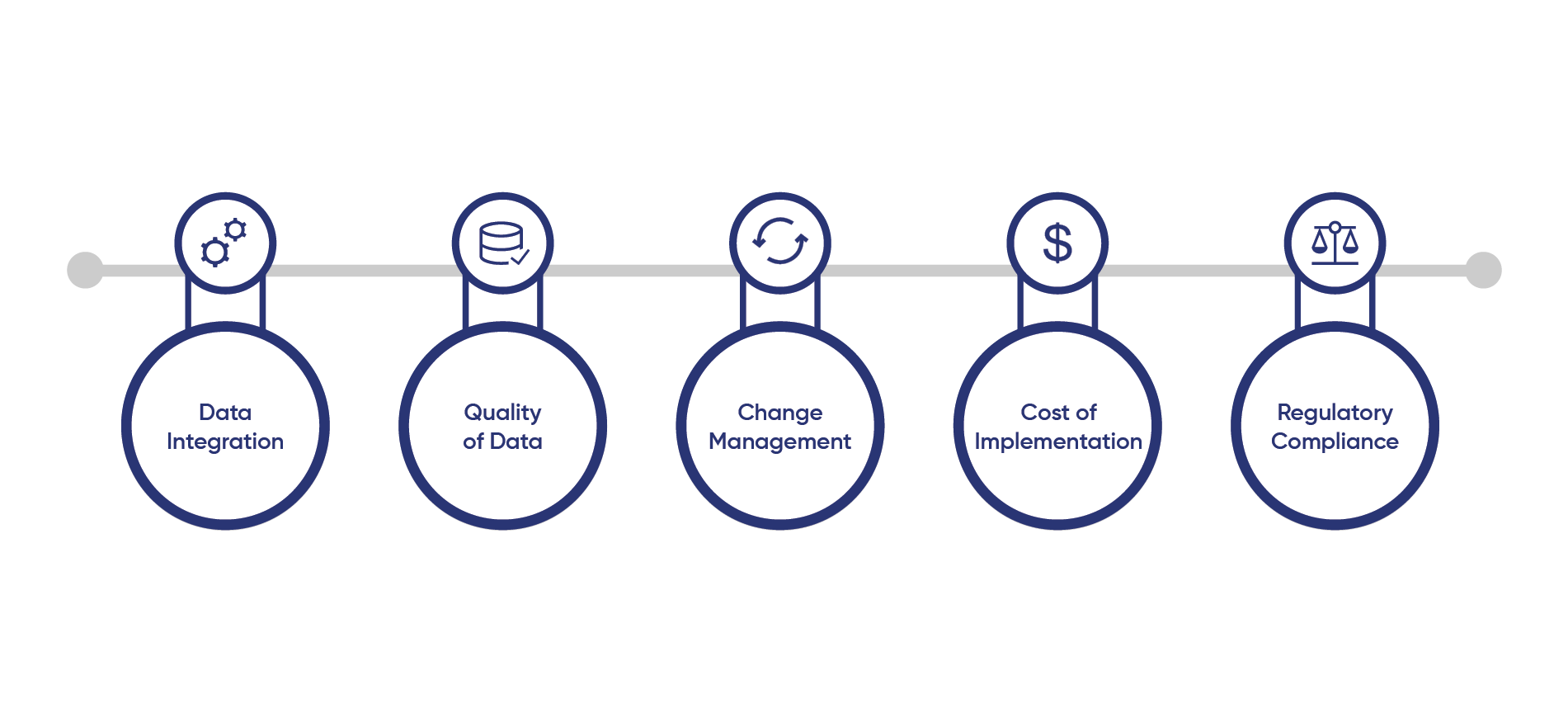
Data Integration
- Solution: Implementing a unified data platform that consolidates information from various sources can help streamline this process.
Quality of Data
- Solution: Regular audits and validation processes should be established to ensure data integrity before it is fed into the AI system.
Change Management
- Solution: Comprehensive training programs should be implemented to help employees understand how to work alongside AI systems effectively.
Cost of Implementation
- Solution: Manufacturers should conduct a thorough cost-benefit analysis to understand long-term savings derived from increased efficiency and reduced disruptions.
Regulatory Compliance
- Solution: Engaging legal experts during the design phase can help navigate regulatory requirements effectively.